The COVID-19 pandemic made healthcare data management difficult and overwhelmed existing IT infrastructure. Making data accessible in desired formats—after sourcing, refining, and transforming—adds more load to the infrastructure. To mitigate these challenges, CIOs need to take a fresh approach to data management, whether that’s through an on-premises storage system, the cloud, or a hybrid model.
Since the pandemic, healthcare CIOs have been focusing more on enterprise data and less on enterprise EMR. Enterprise data not only allows organizations to maintain medical records but also helps derive insights from those records in real-time to improve outcomes.
How have data models evolved in the healthcare industry?
For the past decade, healthcare organizations have been relying on traditional data models. However, traditional data integration processes and governance are no longer sufficient. Reliable, compliant, and accessible data is now crucial to any healthcare organization’s digital transformation.
Most health IT systems work with multiple integrated analytics vendors. In the integration process, data must be transformed to fit into an enterprise data model via binding. There are three binding processes—app-specific, late, and early binding—which differentiate how data models are built.
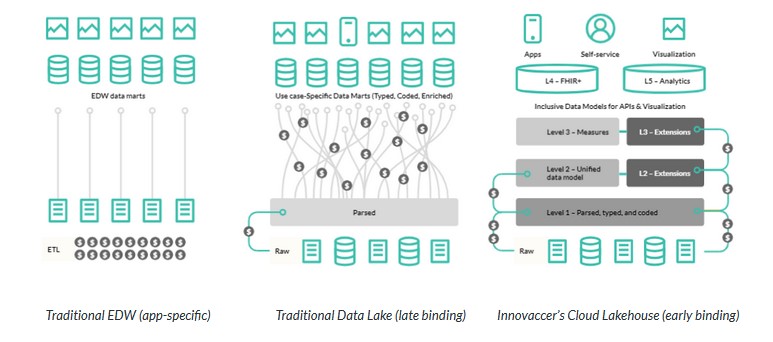
Traditional enterprise data warehouses (EDWs) leverage app-specific binding, meaning they require extensive ETL (extract, transform, and load) work to build a data warehouse. An EDW establishes dedicated subsets—known as data marts—for any business line or department. The processing logic (or ETL logic) is set up to ingest data into data marts anchored by specific formats. The ETL logic needs to be reconfigured every time changes in the source system occur. This is the most common challenge, and it adds more development expenses while building and maintaining a traditional EDW. The ever-increasing cost of development is one of the key factors driving the shift from EDW to data lake models.
The traditional data lake model follows an ETL integration pattern with two separate processes. In one process, raw source data is copied into a data lake; another process transforms data into data marts. Data lake models pose change management issues similar to traditional EDWs, but ETL logic can be reconfigured more efficiently in data lakes than in source systems.
EDW and data lake models bind source data with use case-specific data models with predefined semantics and transformations. Data lakes allow arbitrary storage with no structural or semantic assumptions, and data stored in the lake holds no business value until it’s transformed with an expensive late binding process for each use case. In the long run, data marts increase costs, inefficiencies, and complexities for healthcare organizations due to continuous reconfigurations and poor change management.
Innovaccer’s Cloud Lakehouse eliminates all the challenges of traditional EDWs and data lakes. The Cloud Lakehouse architecture enables transformation by early binding data to its semantics as it is ingested. During the early binding process, source data is rigorously interpreted to give it explicit healthcare context with enforced quality measures. This process eliminates the need to interpret data sources or transform data for new use cases, reducing the costs needed to derive actual business value.
How does Innovaccer’s early binding approach simplify healthcare data journeys?
Healthcare organizations face complex data challenges when delivering care. However, CIOs can swiftly solve these challenges by integrating next-gen smart data technologies with enterprise architecture.
Innovaccer’s Data Activation Platform (DAP) does just that: It transforms complex healthcare data from disparate sources through a six-level process that refines and simplifies data for end-users and seamlessly maps critical patient data into an enterprise architecture. Here’s what the process looks like:
- At Level 0, raw data is captured.
- The data is semantically mapped at Level 1.
- Level 2 represents the core of the platform, i.e., the Unified Data Model (UDM), which acquires, curates, and aggregates healthcare data.
The last three levels involve the restructuring of data based on the parameters defined by the UDM.
- At Level 3, data is formatted with quality scores for enrichment. The quality scores account for regex and column lookup values to fetch data and ensure accuracy.
- The enriched data is automatically harmonized to Level 4 for applications and, lastly, Level 5 for analytics.
There are defined standards for how different types of electronic health data should be exchanged, integrated, and retrieved. Unfortunately, this data isn’t easy for most healthcare professionals to interpret. This is where binding comes in. Mapping data in the early stages while building a data warehouse requires defined business rules—such as admission, discharge, and transfer (ADT)—to maintain uniform data. Innovaccer’s UDM allows most healthcare data to be translated into simplified formats for healthcare professionals.
Organizations need to develop new solutions to fill the gaps in today’s patient care journeys. The extensibility of Innovaccer’s UDM allows organizations to build applications on top of refined data with APIs. For example, if a user wants to leverage Level 2 data with custom elements and tables, the enriched data from the last three levels can be customized according to the user’s specific needs.
Accelerating digital transformation with Innovaccer
Early binding can help healthcare organizations gain more control over their data. Innovaccer’s DAP, UDM, and Cloud Lakehouse architecture are dedicated to accelerating digital transformation for healthcare organizations with foundational and advanced data capabilities. Rigorous early binding in the first few stages of the six-level process offers self-service capabilities to drive innovation and ensures a lower total cost of ownership. However, mapping data may not be the final goal for organizations, and they can also use Innovaccer’s solutions to leverage raw data to gain better insights, build applications for specific uses, and enable visualization tools for advanced reporting and analysis—all of which improves clinical, financial, and operational outcomes.